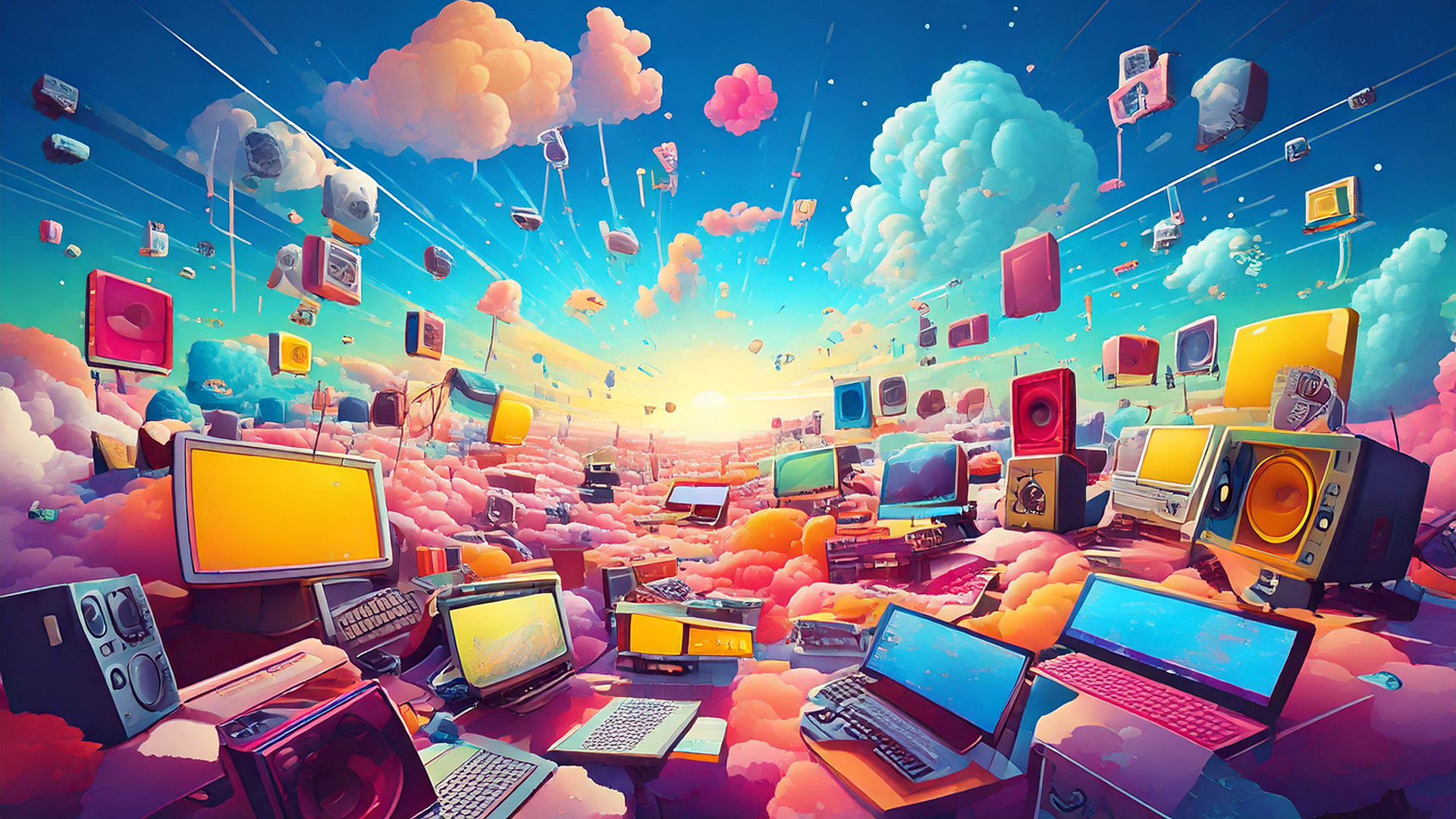
Beyond Text: Multi-Sensory Inputs for Smarter AI-Driven Solutions
By Jake Miller | October 16, 2024
Businesses constantly seek ways to leverage artificial intelligence (AI) to automate tasks, drive insights, and make smarter decisions. However, much of the current AI conversation revolves around text-based data—documents, emails, reports, and spreadsheets. While these inputs have fueled many successful AI applications, they represent only a fraction of the data organizations possess. To truly unlock the potential of AI, we need to move beyond text and embrace rich data sources such as video, audio, telemetry, and sensor data.
It’s crucial for businesses to shift their thinking from traditional field mapping of structured data to integrating diverse data types as direct inputs into generative AI systems. By doing so, companies can enable AI to analyze, contextualize, and make more informed decisions, leading to more personalized, efficient, and accurate outcomes.
The Untapped Potential of Non-Text Data
Many businesses are already collecting massive amounts of video, telemetry, and sensor data, but only a fraction of this data is ever analyzed or used. The sheer volume of unstructured data can be overwhelming, but it also represents an enormous opportunity for AI to drive actionable insights.
• 80% of organizational data is unstructured, according to Gartner, meaning it doesn’t fit neatly into traditional databases or spreadsheets.
• Cisco estimates that by 2025, 82% of all internet traffic will be video, reflecting the growing importance of visual data in today’s digital landscape.
• Forrester reports that less than 10% of companies are effectively analyzing their vast stores of video and visual data.
This untapped resource holds incredible value, and generative AI systems designed to process multimodal data can bridge this gap, providing real-time insights and automation from data streams that were previously overlooked.
Moving Beyond Field Mapping: Embracing Rich Data for Generative AI Solutions
Historically, AI systems have relied on structured data—data organized in fields, tables, and databases. A lot of effort goes into mapping these fields between systems, ensuring the right data ends up in the right place. This structured approach has been effective for certain use cases but is inherently limited in scope. It often overlooks the unstructured, complex data that exists in videos, images, audio recordings, and machine telemetry.
For example, imagine an application that leverages cameras to capture and stream real-time information about road defects, such as potholes, deteriorating lane lines, or other infrastructure issues. This video input, when combined with data from other systems like weather forecasts (to assess how conditions may worsen damage), traffic flow data (to prioritize repairs on heavily trafficked roads), and municipal maintenance schedules (to optimize repair timing and resource allocation), can provide a comprehensive view of the situation. By integrating these data sources, AI can help cities and transportation departments make faster, smarter decisions about which repairs to prioritize, how to allocate crews and materials, and when to schedule the work to minimize traffic disruption. This results in more efficient road maintenance, cost savings, and safer driving conditions.
To unlock the full power of AI, we need to move beyond field mapping and think about how different types of data—especially video and real-time feeds—can serve as direct inputs to solve complex problems. This shift enables AI systems to process and understand live, unstructured data, generating far richer insights and more context-aware decisions.
For instance, a hospital can use text-based medical records to track patient medications, but adding real-time video feeds from patient rooms allows the AI to assess patient movement, posture, and overall condition—making it easier to identify fall risks before they happen. Similarly, combining telemetry data from IoT devices with live video in a factory enables predictive maintenance, preventing machinery breakdowns by spotting early signs of malfunction.
Distinguishing Multimodal Generative AI from Traditional Use of Multi-Format Data
Before diving deeper, it’s important to clarify the distinction between multimodal generative AI and the general use of multiple data formats in traditional AI workflows. Both approaches leverage rich data, but their core methods and purposes differ.
General Use of Multi-Format Data
Traditionally, businesses have used AI to integrate and analyze various types of data—such as text, video, and sensor readings—but in isolation. For example, in a retail environment, sales data might be analyzed separately from in-store camera footage. Each type of data is processed independently, and then the results are correlated after the fact to inform decision-making. While this approach has proven useful, it doesn’t fully leverage the power of these rich data sources because they are treated as discrete inputs rather than being integrated into a unified context.
Multimodal Generative AI
Multimodal generative AI goes further by simultaneously processing multiple data types—text, video, audio, and telemetry—in a cohesive, unified model. Rather than analyzing each data source independently, these systems fuse diverse inputs to create a holistic understanding of the situation. This allows AI to generate more accurate insights, responses, and actions based on the complete picture.
For example, in a healthcare setting, multimodal generative AI could combine patient records (text), real-time video of the patient (visual), and telemetry from wearable devices (sensor data) to dynamically assess the patient’s risk of falls or health deterioration. By integrating these data streams into a single model, the AI is able to generate insights and trigger immediate, contextually appropriate actions—something traditional systems struggle to achieve with isolated data streams.
Not Just Pre-Made Videos: Leveraging Real-Time Streaming Data
When discussing the power of video in AI applications, it’s easy to focus on pre-recorded content like training videos, sales call recordings or customer service interactions. These types of videos are indeed valuable for training AI models or analyzing specific situations after the fact. However, an easily overlooked real breakthrough comes from the ability to process live, streaming data from cameras and sensors in real-time.
Organizations are increasingly adopting video feeds for security, monitoring, and performance analysis, but the vast majority of this data goes unused. AI can unlock the potential of these real-time streams by analyzing them continuously and contextualizing the data in relation to other inputs like telemetry, text, and sensor readings.
For example:
Insurance: Automated Damage Assessment:
Insurance companies can use video data from drones or roadside cameras to assess damage to properties or vehicles after natural disasters like hurricanes, floods, or fires. Drones can capture real-time video footage of affected areas, and AI can analyze the extent of damage to homes, roads, or cars. This video data, when combined with historical claims data and weather data, can help insurers quickly estimate costs and expedite claim approvals without requiring in-person assessments. Since the focus is on infrastructure and not individuals, privacy concerns are minimized.
Finance: ATM and Branch Monitoring:
Banks and financial institutions can use video feeds from ATMs and branch locations to monitor the functioning of equipment, detect vandalism, or identify potential fraud (e.g., tampering with machines). This video can be combined with transaction data and maintenance logs to improve security and ensure operational efficiency. The focus here is on equipment and security, not individuals, so privacy concerns are addressed through anonymized or non-personal footage aimed at protecting the bank’s infrastructure and detecting equipment failures before they affect customers.
Insurance: Roof Damage Inspection with Drones
Insurance companies can deploy drones equipped with cameras to inspect roofs for damage after storms, heavy winds, or hail. These drones capture high-resolution video of shingles, gutters, and structural elements, allowing AI systems to analyze the footage for cracks, missing tiles, or leaks in real-time. When this video data is combined with weather data (e.g., wind speeds, storm intensity), historical claims data (to predict likely damage patterns based on similar past events), and building materials data (to assess how different materials hold up against certain weather conditions), it provides a more powerful, comprehensive assessment of the damage. This contextual information helps insurers make quicker and more accurate decisions about repair estimates, reduce the need for on-site visits, and ensure claims are processed more efficiently—all while minimizing privacy concerns, as the focus remains on structures, not people.
Unlocking the Full Potential of AI with Rich Data
As we move into a future increasingly driven by AI, businesses must recognize the value of going beyond text. The ability to integrate and analyze rich, multimodal data—especially real-time streaming video, audio, and telemetry—unlocks new levels of intelligence and automation for workflows.
Executives seeking to stay competitive need to think about multimodal generative AI as a way to truly harness the vast amounts of unstructured data within their organizations. From healthcare to retail to manufacturing, this approach enables more personalized, accurate, and contextually aware decision-making that will shape the future of AI-driven business.
The future is rich with data. The question is: are you ready to unlock its full potential?